A machine learning method for predicting telescope cycle time
Artificial intelligence is predicting duration of observations at OCM, helping with scheduling and optimization.
A machine learning method for predicting telescope cycle time applied to the Cerro Murphy Observatory
M. Kicia, M. Kałuszyński, M. Górski, R. Chini, G. Pietrzyński
The telescope cycle time estimation (prediction) is one of astronomy’s instrumental issues, which accompanies every astronomer in their daily work, primarily for observation scheduling. Accurate time scheduling is becoming more important in today’s increasingly automated, fully or partial robotic observatories. There are many situations in which timely observations are crucial, e.g. observations of eclipsing binaries, pulsating stars. Therefore, it is crucial to place an observation accurately in order not to miss a specific object phase or the event altogether.
The authors present a fully automatic software that works without any user intervention. The software is based on the Machine Learning method, specifically a polynomial multiple regression method, and trained on the real data from the telescopes of the Cerro Murphy Observatory (OCM). Error evaluation shows that for 84 % of nights, the prediction error in operation time prediction does not exceed 2% (i.e. 12 minutes in for a 10-hour observing night).
The software is already working at OCM and optimizes the efficiency of the observations but can also be used at any other observatory. The code is available on request from the corresponding author.
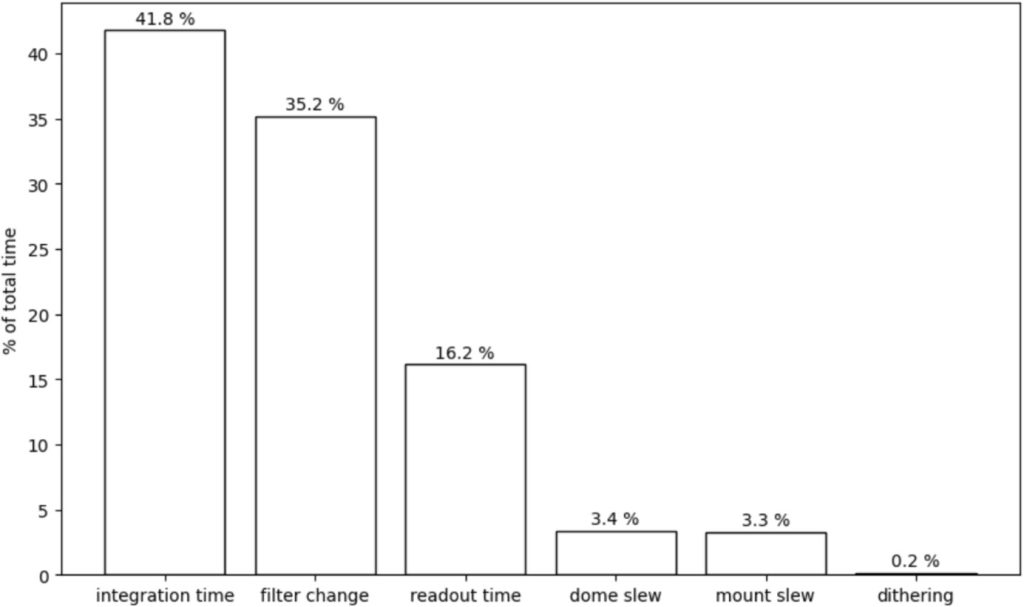
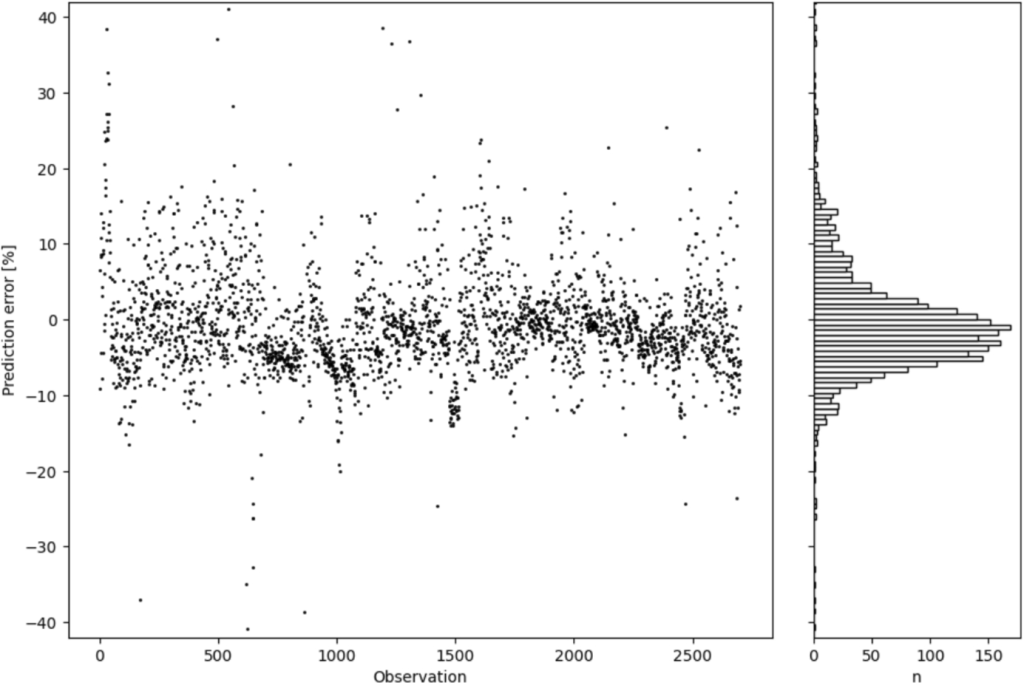